A number of industries are now employing Generative AI (Gen-AI) to accelerate the efficiency of their processes. As a result of the AI revolution (heightened by the public prominence of bots like Chat GPT and others), many companies around the world across a range of different functional areas (including supply chains, marketing, product making, research, analysis, and more) have chosen to integrate at least some form of AI into their operations.
Indeed, the sheer scale of the AI industry has ballooned over the course of the last year as several sectors have raced to integrate this transformative new tech.
According to Next Move Strategy Consulting, the AI market is predicted to increase from nearly $100 billion 2023 to almost $2 trillion by 2030. Meanwhile, TechJury reports that 35% of companies are currently using AI, while a further 42% are now exploring its potential implementation in the near future.
Companies shouldn’t choose to implement Generative AI without accessing the actual quality and meaning of their data first.
They need to consider the security of the data, since open Generative AI tools will be using it to make decisions, and these tools can be used by their competitors. They also need to bear in mind how the potential answers provided by AI can sometimes be inaccurate, and there is often little or no chance for human users to determine where their answers have come from.
Because of this, companies looking to use Generative AI have to rely on forms of data architecture that can provide the users of this technology with the skills and time needed to implement their solutions internally.
So, how can enterprises safely and effectively integrate Generative AI?. There are currently two architectures for integrating Generative AI: data mesh vs. data fabric. Choosing which option is best for an enterprise can be challenging for Heads of Data and IT — especially if they’re still getting to grips with this groundbreaking new tech.
With all that in mind, this article will examine each integration method in detail to help decision-makers starting on their Gen-AI journey make the right choice for their individual business needs.
What is a Data mesh?
Before we go any further, we need to establish what the term ‘data mesh’ actually means in the context of a Gen-AI implementation.
According to Gartner, data mesh is an architectural approach driven by federating data management responsibilities through distributed data governance. The data mesh architecture is a decentralized approach to data that allows domain teams to perform cross-domain data analysis on their own. It is designed to make the data involved in AI integration more accessible to all stakeholders, such as the owners, producers, and consumers of data.
Through the data mesh approach, domain teams ingest operational data and build analytical data models as data products to perform their own analysis and publish data products with data contracts to serve the needs of other domains.
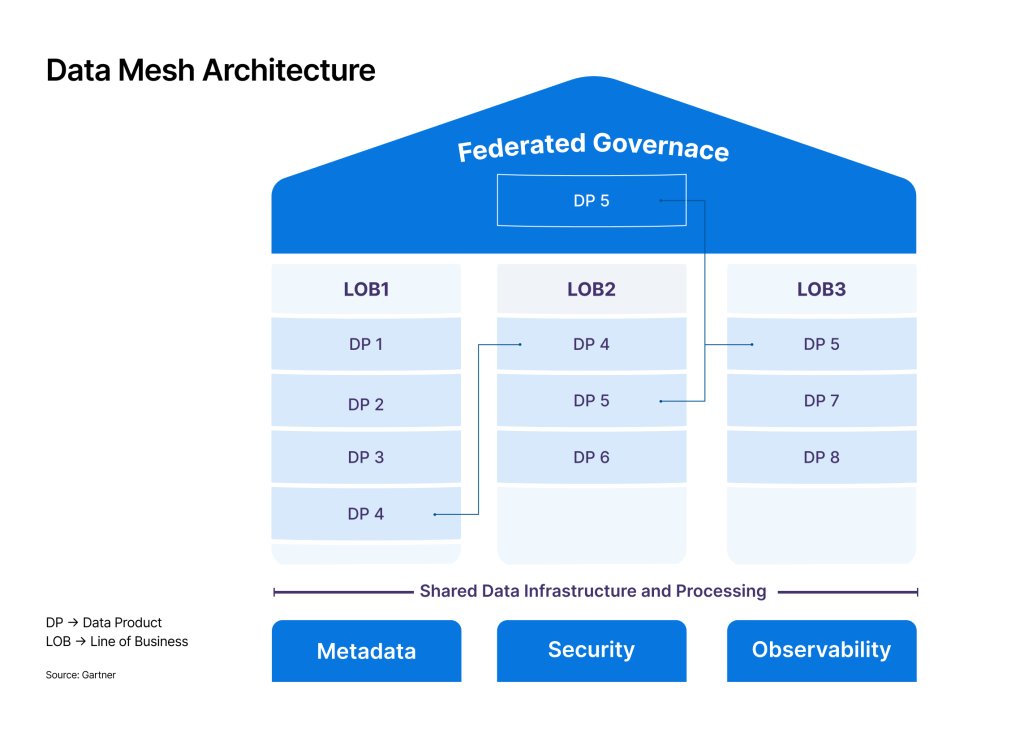
Benefits of data mesh
Bearing this in mind, what are the key benefits of this architecture? A few advantages include:
- Utility: Data mesh can provide greater control and ownership of datasets from the producers themselves.
- Relevancy: The mesh helps reduce complexity by allowing users to access only relevant information instead of all available datasets.
- Speedy Response Time: Organizations can also respond quickly to changing business needs by making adjustments on-the-fly with minimal disruption of operations.
- Scaling: The use of a data mesh means that teams attempting to integrate their Gen-AI can scale up or down depending on demand without compromising performance or reliability.
Drawbacks of data mesh
However, it’s important to bear in mind that there are some disadvantages to a data mesh architecture, such as:
- Complexity: Implementing a data mesh can be complex, requiring several operational components, such as databases, APIs, messaging systems, and other technologies, to function correctly.
- Cost: A data mesh requires a heavy internal investment in hardware and software resources, such as setup costs and ongoing operational expenses, like storage and licensing fees
- Interoperability: Issues with this can arise if several organizations use incompatible product versions. This means that they will be unable to exchange information effectively, which can lead to project delays or miscommunications between the various stakeholders.
For companies who might be discouraged from the data mesh architecture because of these drawbacks, there is a much better way for them to achieve their goals with a different architecture entirely: data fabric.
The value of data fabric
What is data fabric?
In contrast to data mesh, data fabric automatically or semi-automatically creates a single, interoperable environment that connects all the different data and analytics elements in an organization’s ecosystem, utilizing metadata and enabling actionable data governance.
Data fabric provides a single point of access to all data sources. The fabric leverages data integration, active metadata, knowledge graphs, profiling, ML, and data cataloging, ensuring data is consumable across multiple use and re-use cases.
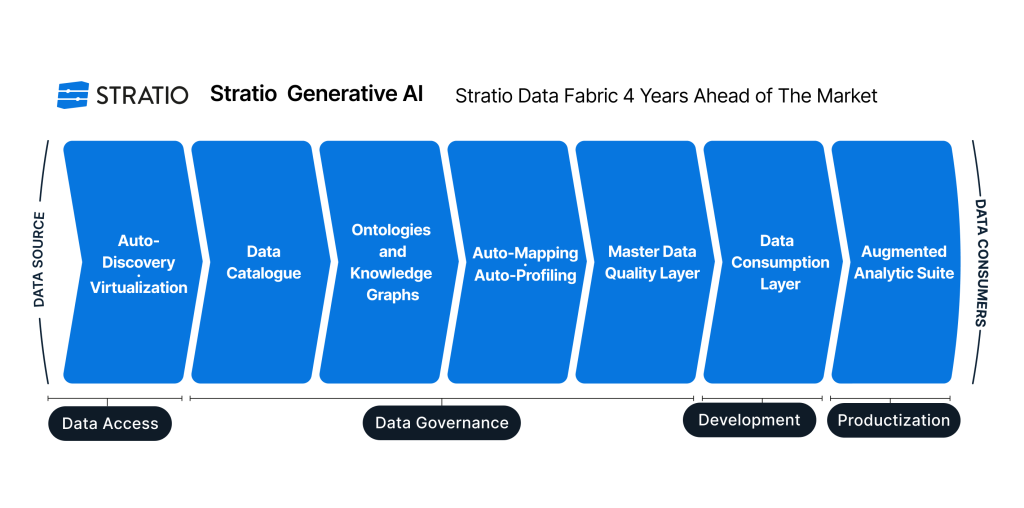
Benefits of data fabric
Data fabric takes a unique approach to integrating data sources by using metadata to create a virtualized data layer, avoiding moving data from its storage location and preserving data governance. It then adds semantic knowledge (business and industry ontologies) for context and meaning, and enriched data models.
This eliminates costly, time-consuming, error-prone custom integration projects and reduces maintenance over time. It gives data meaning for easy consumption by the business users.
Data fabric product can provide enterprises with a comprehensive view of all data, whether it stems from the cloud, on-premise, or multiple other sources and formats — making it all accessible from a single interface. Once all data is available, quality and governance rules can be automatically applied as well as knowledge graphs and ontologies, enabling data marketplaces for business users or industrialization of the AI models.
Lastly, data fabric guides implementation of Generative AI, because the data in data fabric is already secure, has high quality and business meaning, making it easy to plug in any generative AI tool, including purpose-built generative AI for enterprise.
This means that employees across the organization can contribute to the AI’s ongoing development, building its intelligence and utility with the appropriate data. They can ask questions in natural language and receive insights, answers, reports, references to processes and procedures, and much more in a matter of seconds.
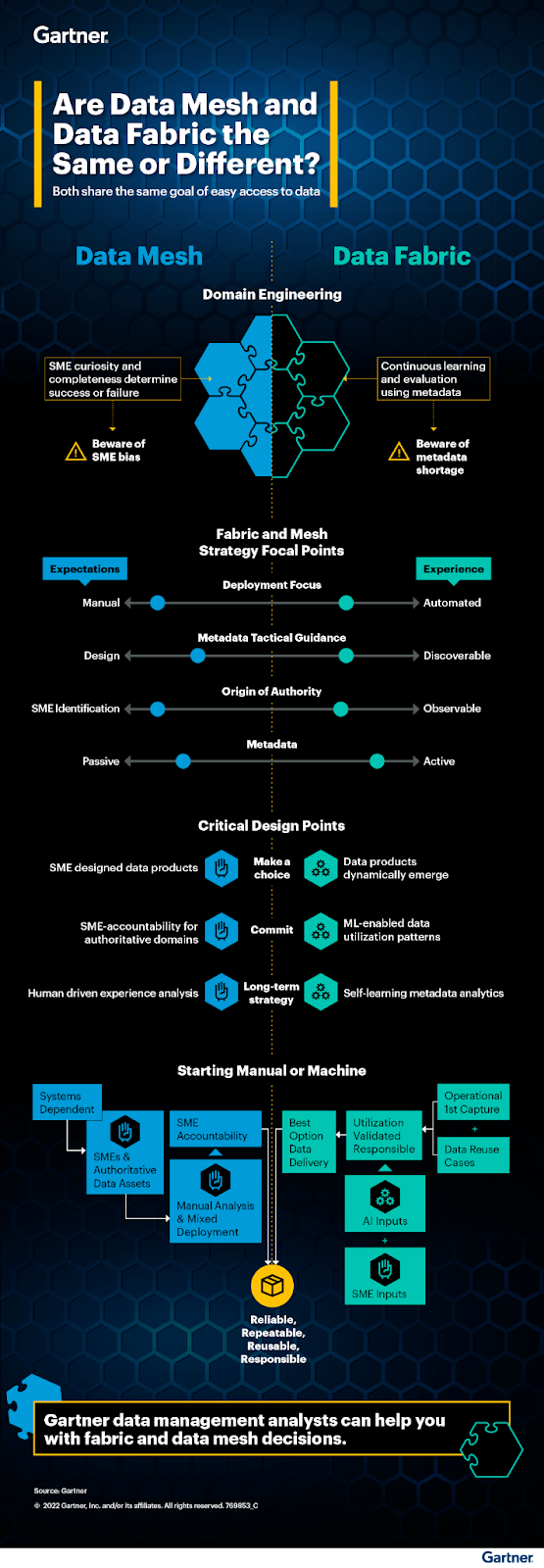
The power of Stratio Generative AI Data Fabric
When it comes to the issue of data mesh vs. data fabric, we find that data fabric comes out on top for companies looking to implement Gen-AI. And it’s not just the opinion of our 50+ enterprise clients: Gartner also believes that data fabric is the only way forward for data management in the next ten years.
Stratio is a market leader in the data management space, and our end-to-end Generative AI Data Fabric product is the first of its kind in the world. Statio’s Gen-AI Data Fabric is the best solution for companies looking for an intuitive and effective AI interface. It fully automates data access and governance, security, and quality at an enterprise level and at scale.
So, if you’d like to learn how Stratio can help your team get the most out of its data, then visit our website to read our latest whitepaper, or you can learn more about the data management space at our blog here.